Deep Dive Perception Module
This page aims to give details into the concepts of MOSAICs perception module, for information on the usage view the documentation here. Perception in MOSAIC is evaluated in a two-step process:
- First a spatial search, using a spatial index, is performed to get a pre-selection of relevant vehicles
- Second a Field-Of-View Filter is used to get a list of actually perceived vehicles
Spatial Search & Spatial indexes
A spatial index is a data structure representing simulation entities on a 2D-plane allowing for fast spatial searches.
For our purpose this means, that we create a minimum bounding rectangle for a vehicles' perception range and perform a
spatial search for entities in that rectangle.
To allow every vehicle to access the spatial index we opted for a global implementation in the
Application Simulator, which is only updated when some vehicle
requests a spatial search (lazy loading).
MOSAIC provides two implementations for the spatial index: tree
, and grid
.
Quad-Tree
The Quad-Tree index represents entities in a tree data structure, that stores entities in a node up to a splitSize
amount.
Every node strictly has no or four children. The four children divide the space into four equally sized quadrants.
Additionally, a joinSize
and a maxDepth
are configured, that control, when four children are joined back to one node and
the maximum depth of the tree respectively.
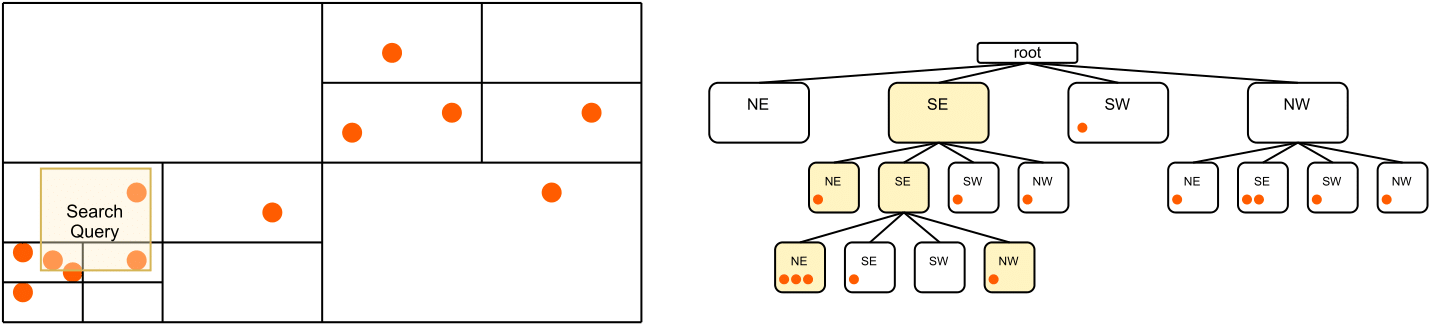
The Quad-Tree has the advantage of dynamically separating the space according to the utilization of the map. However, in practise we measured slightly worse performance compared to the grid.
Grid
The grid is a simple data-structure that divides the space into equally sized cells with configured cellWidth
s and cellHeight
s.
Entities positions are converted to grid-coordinates and stored in a map.
When doing a spatial search the pre-selection of vehicles happens by returning all entities in cells, that overlap with the search area.
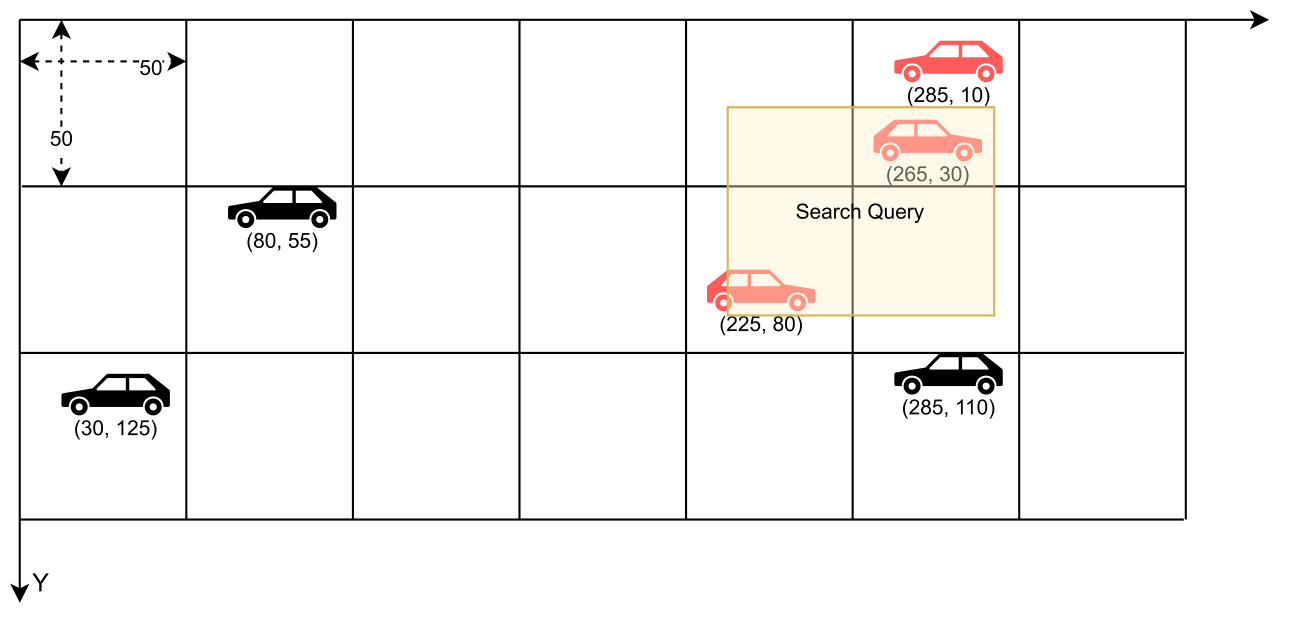
While showing slightly better performance than the Quad-Tree, the grid requires the memory allocation of all cells at start-up,
which can lead to large memory consumption in big scenarios.
Additionally, the optimal grid size depends on the viewing ranges of your entities. In the best case scenario a maximum of four grid-cells
have to be queried. This is the case if the cellWidth
and cellHeight
are at least the size of your longest viewing range.
Field-Of-View Filter
After a pre-selection of entities has been made using the spatial index we have to evaluate if vehicles are actually in the field-of-view of the ego vehicle. The field-of-view boils down to the sector of a circle, which is defined by its two bounding vectors $\overrightarrow{b}$ and $\overrightarrow{c}$ and the radius/sight distance $h$. Using the dot product, we can figure out if an object lies to the right or the left of a vector. Combining this with a check if the distance is smaller than $h$ allows us to determine whether an object $\overrightarrow{m}$ lies within the perception range.
$$ \begin{align} &\text{1. }\overrightarrow{c} \cdot \overrightarrow{m} \geq 0 \\ &\text{2. }\overrightarrow{m} \cdot \overrightarrow{b} \geq 0 \\ &\text{3. }|\overrightarrow{m}| \leq h \end{align} $$
Right: Field-Of-View evaluation ($\overrightarrow{m}$ is within range, $\overrightarrow{n}$ is not)